Data Quality: The Foundation of Reliable Analytics and AI
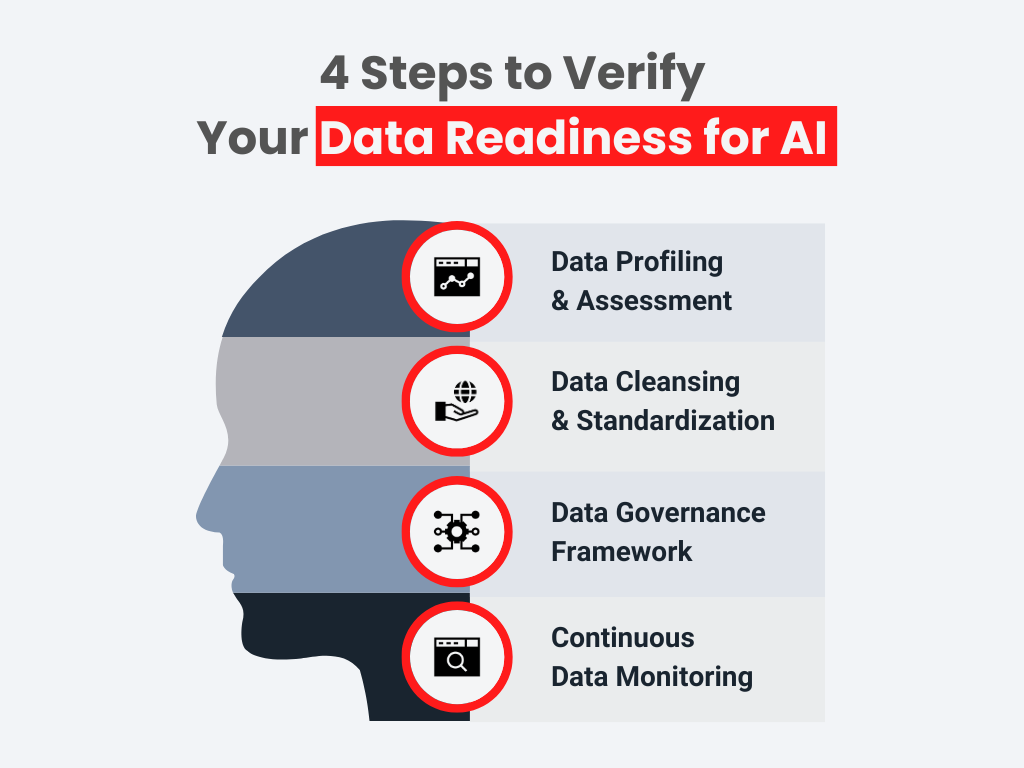
In the age of big data, organizations are constantly seeking to leverage analytics and artificial intelligence (AI) to drive decision-making, optimize operations, and uncover new opportunities. However, the accuracy, reliability, and cleanliness of your data are the key foundations for effective analytics and successful AI implementations.
Data quality plays a pivotal role in ensuring that the insights derived from analytics and AI are trustworthy, actionable, and valuable. Without high-quality data, even the most sophisticated AI algorithms and analytical tools will produce misleading, inconsistent, or flawed results.
📊 What is Data Quality?
Data quality refers to how well your data serves its intended purpose—whether it’s for analysis, decision-making, or machine learning applications. It encompasses several factors, including:
- Accuracy: Is the data correct and free of errors?
- Completeness: Does the data include all necessary information?
- Consistency: Are there no contradictions or discrepancies in the data?
- Timeliness: Is the data up to date and relevant to the current context?
- Relevance: Does the data align with the goals of the analysis or AI model?
- Validity: Is the data properly formatted and usable?
🚀 Why Data Quality Matters for Analytics and AI
✅ 1. Improves Accuracy of Insights
Analytics and AI depend on data to generate insights. Poor-quality data leads to false conclusions, making it difficult for organizations to make informed decisions or predict outcomes with confidence.
🤖 2. Enhances AI Model Performance
AI models learn from historical data. If the data is inaccurate or incomplete, the model will be trained on flawed information, leading to biased results, lower accuracy, and potentially harmful decisions.
🔄 3. Increases Trust in Decision-Making
Quality data provides a solid foundation for decision-making, helping leaders make more confident, data-driven choices rather than relying on gut feelings or assumptions.
📈 4. Enables Better Forecasting
For predictive analytics and AI to work effectively, data must be consistent and timely. Poor-quality data can distort predictions, leading to misguided strategies and missed opportunities.
🛡️ 5. Reduces Risk and Errors
Poor data quality can lead to regulatory non-compliance, incorrect financial reporting, and operational errors. Ensuring data quality reduces these risks and helps maintain compliance with data protection laws.
🧠 Real-World Example
Consider a retail company that wants to predict customer purchasing behavior using AI. If their customer data is riddled with errors, missing details (like product preferences), or inconsistent across different systems, the AI model will be trained on unreliable information. This could result in incorrect product recommendations, missed sales, and dissatisfied customers.
On the other hand, if the company ensures high-quality data—accurate, complete, and up-to-date—the AI model will be better equipped to provide valuable insights, improving customer experience and driving sales.
🛠️ How i4 Tech Integrated Services Can Help
At i4 Tech Integrated Services, we specialize in helping businesses:
- Improve data quality through cleaning, validation, and standardization
- Implement automated processes for continuous data quality monitoring
- Ensure that data is ready for AI and analytics with proper structuring and formatting
- Provide data governance strategies to maintain data integrity
- Train teams on best practices for maintaining high-quality data